TOP
Early Phase
Work-in-progress
Abstract (Last Update: January 02, 2024)
Abstract (Last Update: Jan. 06, 2024)
Abstract (Last Update: January 02, 2024)
This paper develops a new asset pricing model designed to address the limitations of the Federal Housing Finance Agency's repeat sales method, particularly in accurately measuring housing prices in low-volume trading areas. In classical asset pricing models, the dynamic programming approach assumes a recursive relationship in which decisions are based on state variables from the previous period. While this approach is more reliable with abundant data, limited data can introduce significant bias, as the model may struggle to accurately capture the underlying dynamics in low-sample scenarios. Using Bayesian inference, this paper aims to propose a robust framework that overcomes this bias.
This paper develops a new asset pricing model designed to address the limitations of the Federal Housing Finance Agency's repeat sales method, particularly in accurately measuring housing prices in low-volume trading areas. In classical asset pricing models, the dynamic programming approach assumes a recursive relationship in which decisions are based on state variables from the previous period. While this approach is more reliable with abundant data, limited data can introduce significant bias, as the model may struggle to accurately capture the underlying dynamics in low-sample scenarios. Using Bayesian inference, this paper aims to propose a robust framework that overcomes this bias.
This paper develops a new asset pricing model designed to address the limitations of the Federal Housing Finance Agency's repeat sales method, particularly in accurately measuring housing prices in low-volume trading areas. In classical asset pricing models, the dynamic programming approach assumes a recursive relationship in which decisions are based on state variables from the previous period. While this approach is more reliable with abundant data, limited data can introduce significant bias, as the model may struggle to accurately capture the underlying dynamics in low-sample scenarios. Using Bayesian inference, this paper aims to propose a robust framework that overcomes this bias.
Selected Figures & Tables
Selected Figures & Tables
Selected Figures & Tables
Figures 1.
Stylized Fact: Example
Figures 1.
Stylized Fact: Example
Figures 1.
Stylized Fact: Example
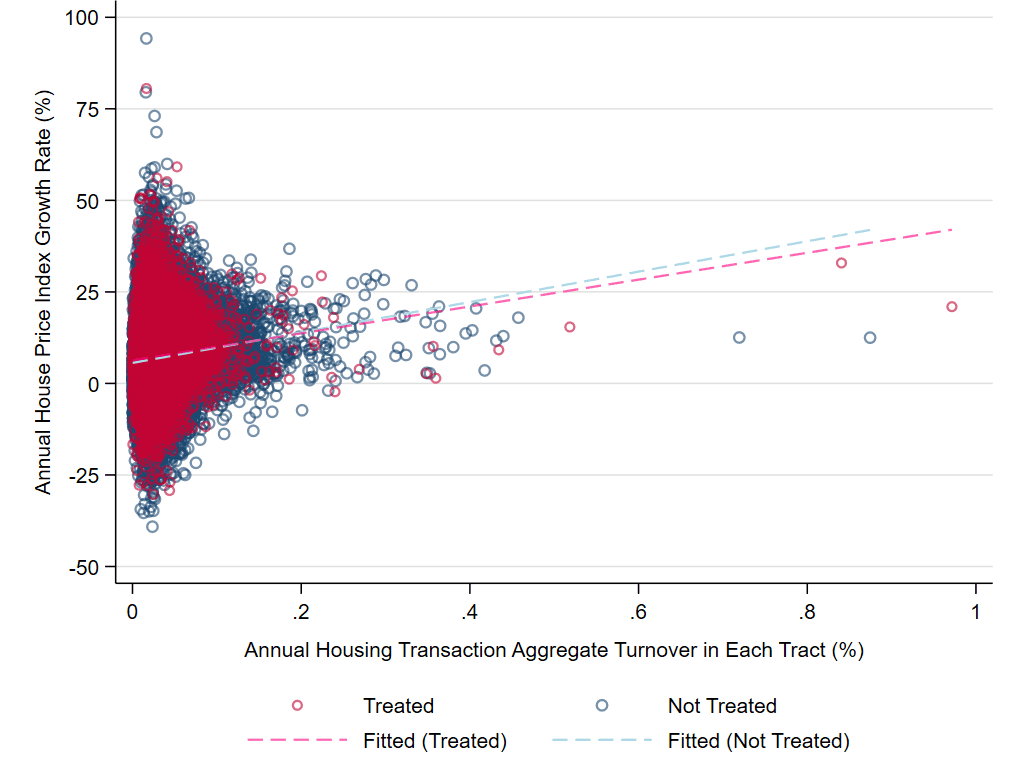
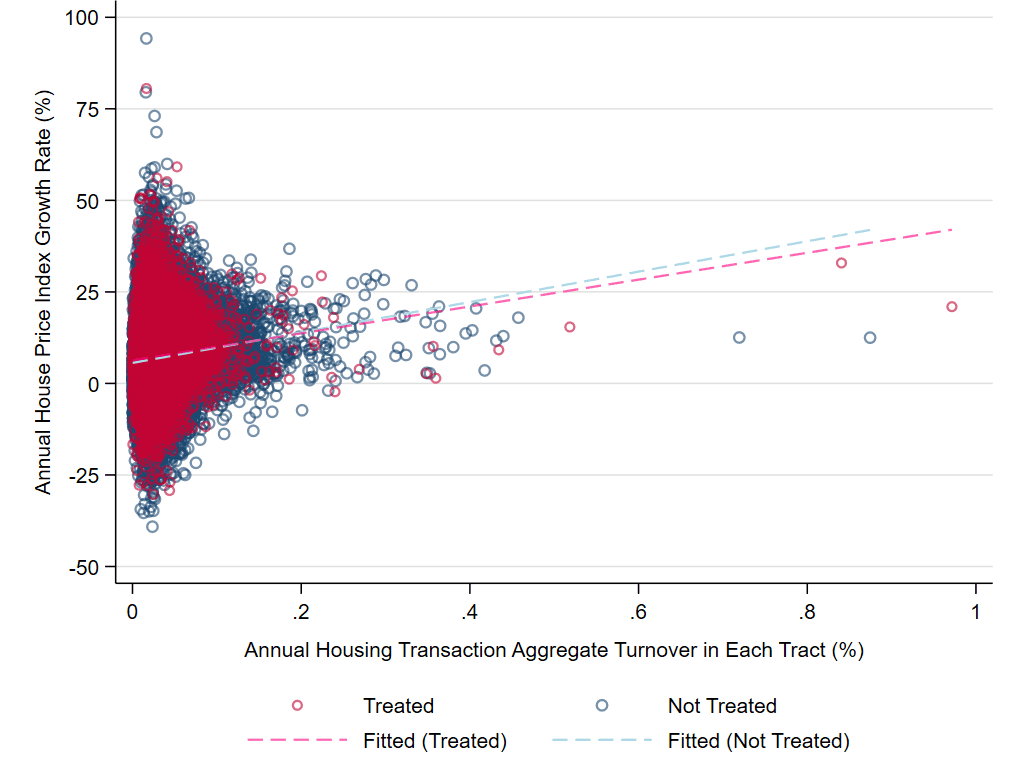
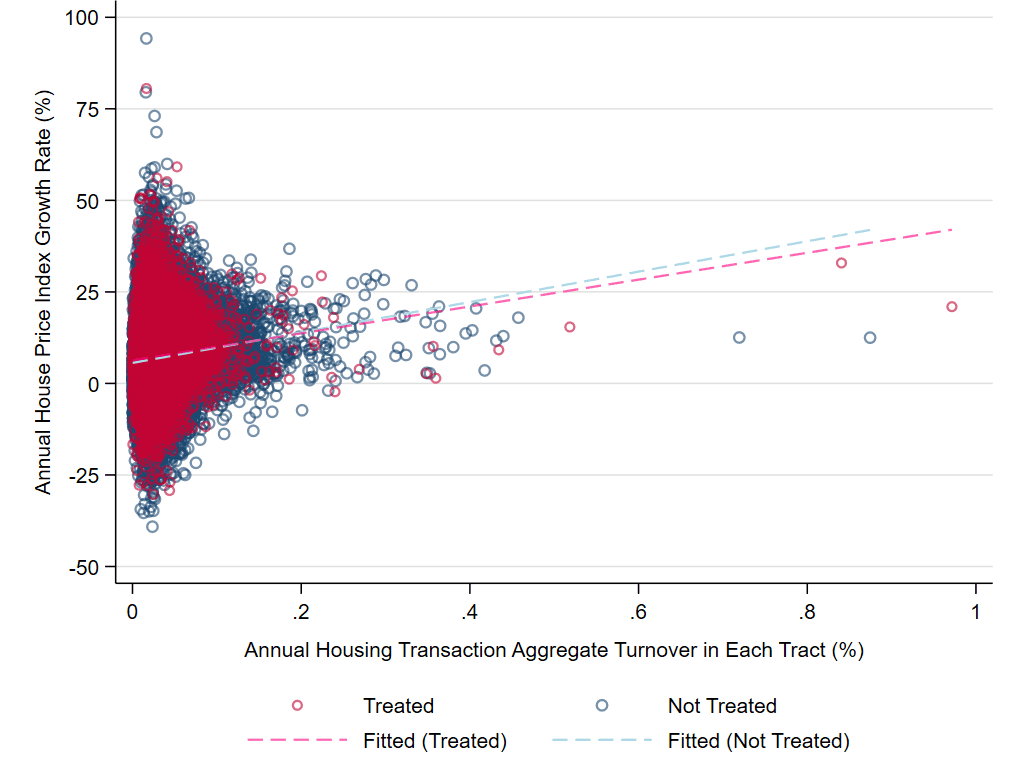
Figure 1 shows the association of annual house price growth rate with the aggregate trading volume turnover between 2014 and 2021 using datasets from the Federal Housing Finance Agency and CoreLogic Tax and Deed data.
Figure 1 shows the association of annual house price growth rate with the aggregate trading volume turnover between 2014 and 2021 using datasets from the Federal Housing Finance Agency and CoreLogic Tax and Deed data.
Figure 1 shows the association of annual house price growth rate with the aggregate trading volume turnover between 2014 and 2021 using datasets from the Federal Housing Finance Agency and CoreLogic Tax and Deed data.
Conclusion
Conclusion
Conclusion
Work-in-progress.
Work-in-progress.
Work-in-progress.
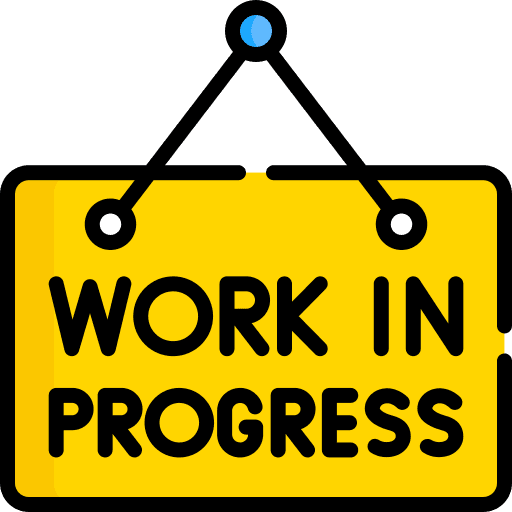
© 2025 WOOSERKPARK.COM. All rights reserved
© 2025 WOOSERKPARK.COM. All rights reserved