TOP
Develop or Distribute?
The Higher Authority's Role
in Fiscal Competition
Develop or Distribute?
The Higher Authority's Role
in Fiscal Competition
Journal (TBA)
Work-in-progress
Abstract (Last Update: April 27, 2025)
Abstract (Last Update: April 27, 2025)
Abstract (Last Update: Apr. 24, 2025)
Court-ordered school finance reform (SFR) has improved the future economic outcomes of low-income students. However, critics often raise concerns about the equity-efficiency trade-off, arguing that the state-mandated SFR, which requires equalization of per-pupil spending, leads to intergovernmental conflict because its binding obligation creates incentives for local governments to prioritize their self-interests, such as regional development, over the general welfare of the state. A representative example is the California legislature's decision to restrict the use of property taxes, which led to a 2011 Supreme Court case that concluded the transfer of assets from local redevelopment agencies to state oversight. Using this natural experiment design with local agency compliance variation in both the size and timing of the asset transfers, I document that higher-level authority can be an effective channel for mitigating fiscal competition, especially when lower levels prioritize their self-interest. State oversight resulted in a positive continuous treatment effect, increasing per-pupil spending by 1.6% and land development growth by 6.8% in school districts. The implication suggests that higher-level intervention can help prevent a "race to the bottom" scenario by reducing competitive fiscal pressures and internalizing negative externalities, thereby promoting both equitable education spending and balanced regional development growth.
Court-ordered school finance reform (SFR) has improved the future economic outcomes of low-income students. However, critics often raise concerns about the equity-efficiency trade-off, arguing that the state-mandated SFR, which requires equalization of per-pupil spending, leads to intergovernmental conflict because its binding obligation creates incentives for local governments to prioritize their self-interests, such as regional development, over the general welfare of the state. A representative example is the California legislature's decision to restrict the use of property taxes, which led to a 2011 Supreme Court case that concluded the transfer of assets from local redevelopment agencies to state oversight. Using this natural experiment design with local agency compliance variation in both the size and timing of the asset transfers, I document that higher-level authority can be an effective channel for mitigating fiscal competition, especially when lower levels prioritize their self-interest. State oversight resulted in a positive continuous treatment effect, increasing per-pupil spending by 1.6% and land development growth by 6.8% in school districts. The implication suggests that higher-level intervention can help prevent a "race to the bottom" scenario by reducing competitive fiscal pressures and internalizing negative externalities, thereby promoting both equitable education spending and balanced regional development growth.
Court-ordered school finance reform (SFR) has improved the future economic outcomes of low-income students. However, critics often raise concerns about the equity-efficiency trade-off, arguing that the state-mandated SFR, which requires equalization of per-pupil spending, leads to intergovernmental conflict because its binding obligation creates incentives for local governments to prioritize their self-interests, such as regional development, over the general welfare of the state. A representative example is the California legislature's decision to restrict the use of property taxes, which led to a 2011 Supreme Court case that concluded the transfer of assets from local redevelopment agencies to state oversight. Using this natural experiment design with local agency compliance variation in both the size and timing of the asset transfers, I document that higher-level authority can be an effective channel for mitigating fiscal competition, especially when lower levels prioritize their self-interest. State oversight resulted in a positive continuous treatment effect, increasing per-pupil spending by 1.6% and land development growth by 6.8% in school districts. The implication suggests that higher-level intervention can help prevent a "race to the bottom" scenario by reducing competitive fiscal pressures and internalizing negative externalities, thereby promoting both equitable education spending and balanced regional development growth.
Selected Figures & Tables
Selected Figures & Tables
Selected Figures & Tables
Figures 1a & 1b.
California School Districts and Tax Increment Financing Districts Maps
Figures 1a & 1b.
California School Districts and Tax Increment Financing Districts Maps
Figures 1a & 1b.
California School Districts and Tax Increment Financing Districts Maps
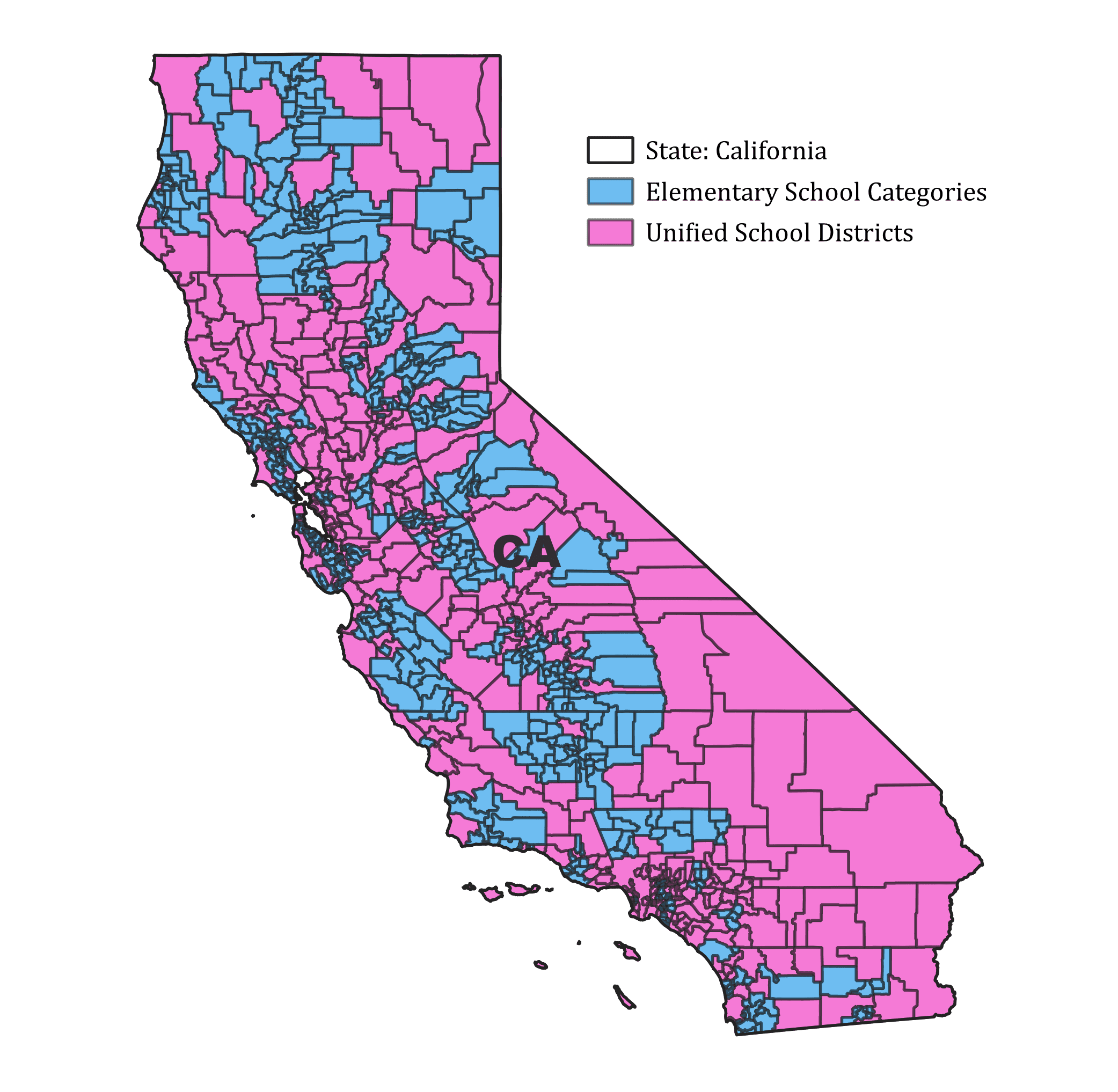
Figure 1a. Census Unified and Elementary School Districts
Figure 1a. Census Unified and Elementary School Districts
Figure 1a. Census Unified and Elementary School Districts
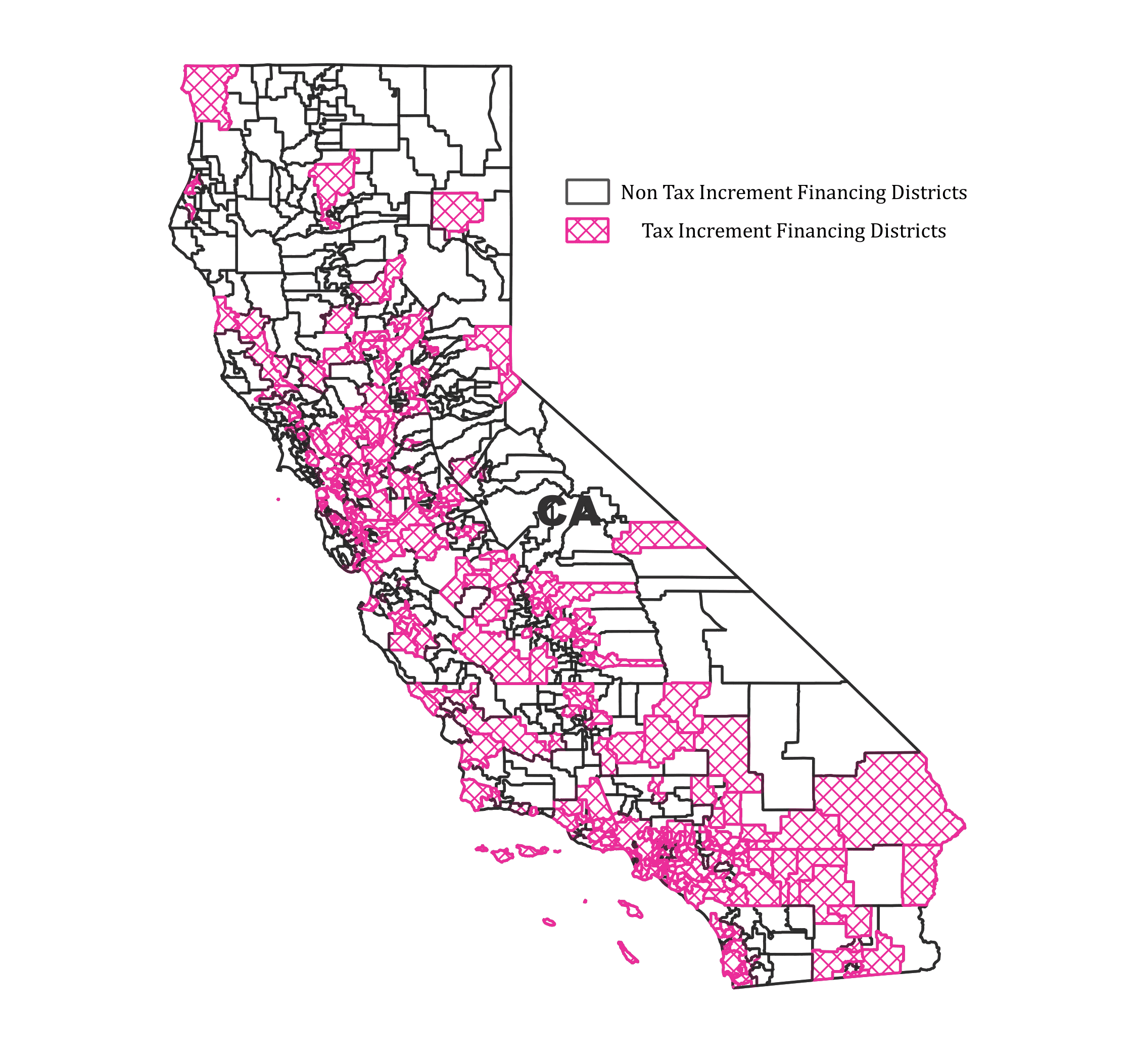
Figure 1b. Tax Increment Financing Districts
Figure 1b. Tax Increment Financing Districts
Figure 1b. Tax Increment Financing Districts
Figure 1a shows a map of California school districts using geocoordinate data from the California Geoportal. The map uses pink to represent unified school districts and blue to represent elementary school categories. As of the 2022-2023 academic year, this dataset includes records for 939 school districts. Figure 1b shows whether school districts used tax increment financing (pink shaded) or not (none).
Figure 1a shows a map of California school districts using geocoordinate data from the California Geoportal. The map uses pink to represent unified school districts and blue to represent elementary school categories. As of the 2022-2023 academic year, this dataset includes records for 939 school districts. Figure 1b shows whether school districts used tax increment financing (pink shaded) or not (none).
Figure 1a shows a map of California school districts using geocoordinate data from the California Geoportal. The map uses pink to represent unified school districts and blue to represent elementary school categories. As of the 2022-2023 academic year, this dataset includes records for 939 school districts. Figure 1b shows whether school districts used tax increment financing (pink shaded) or not (none).
Figures 2.
Successor Agency Locations and Jurisdictions Geographic Boundaries
Figures 2.
Successor Agency Locations and Jurisdictions Geographic Boundaries
Figures 2.
Successor Agency Locations and Jurisdictions Geographic Boundaries

Note: Figure 2 shows the locations of successor agencies (pink dots) and the geographic boundaries of jurisdictions (counties, cities, and school districts) in California.
Note: Figure 2 shows the locations of successor agencies (pink dots) and the geographic boundaries of jurisdictions (counties, cities, and school districts) in California.
Note: Figure 2 shows the locations of successor agencies (pink dots) and the geographic boundaries of jurisdictions (counties, cities, and school districts) in California.
Figures 3a & 3b.
California Students Information
Figures 3a & 3b.
California Students Information
Figures 3a & 3b.
California Students Information
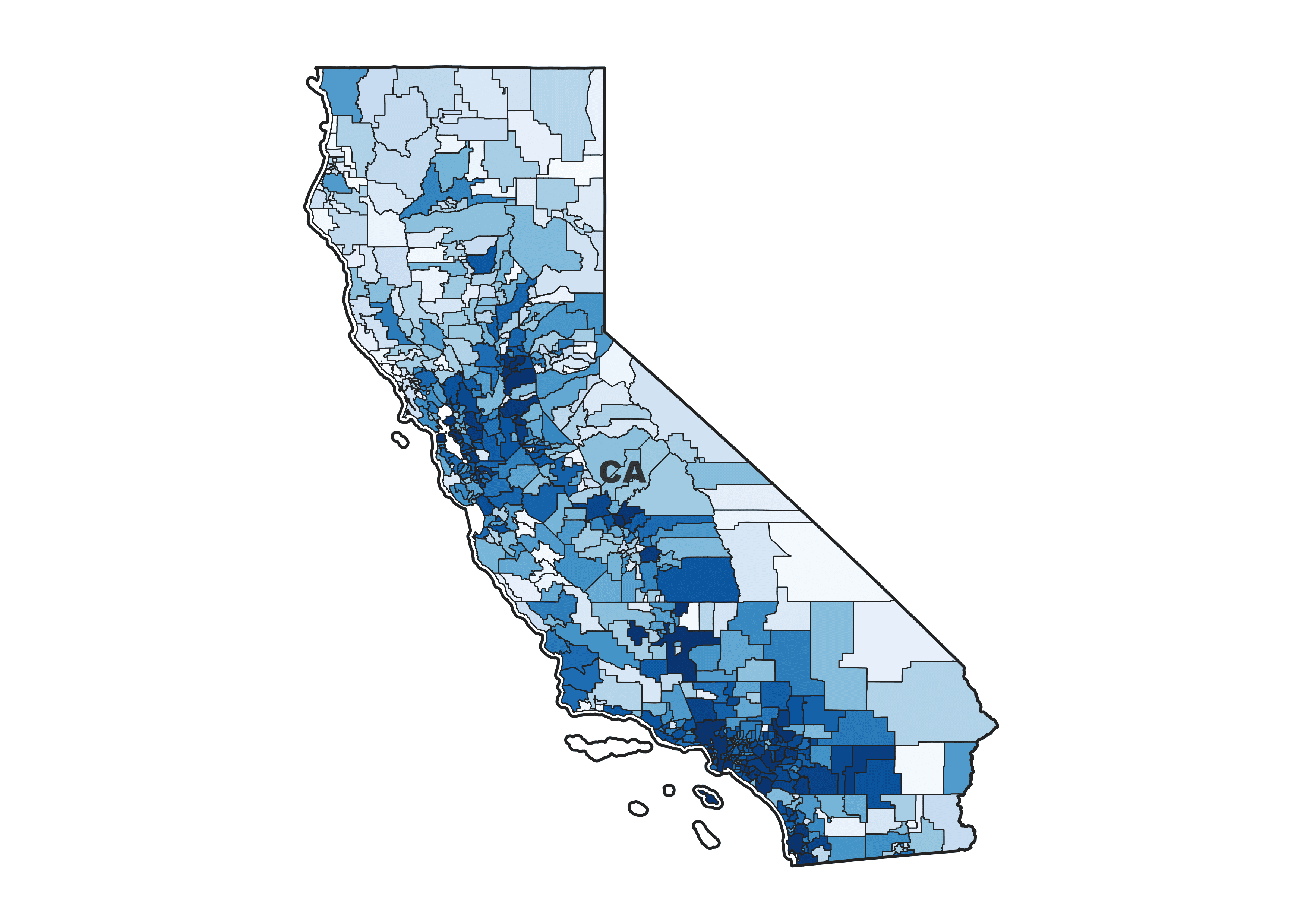
Figure 3a. Total Students
Figure 3a. Total Students
Figure 3a. Total Students
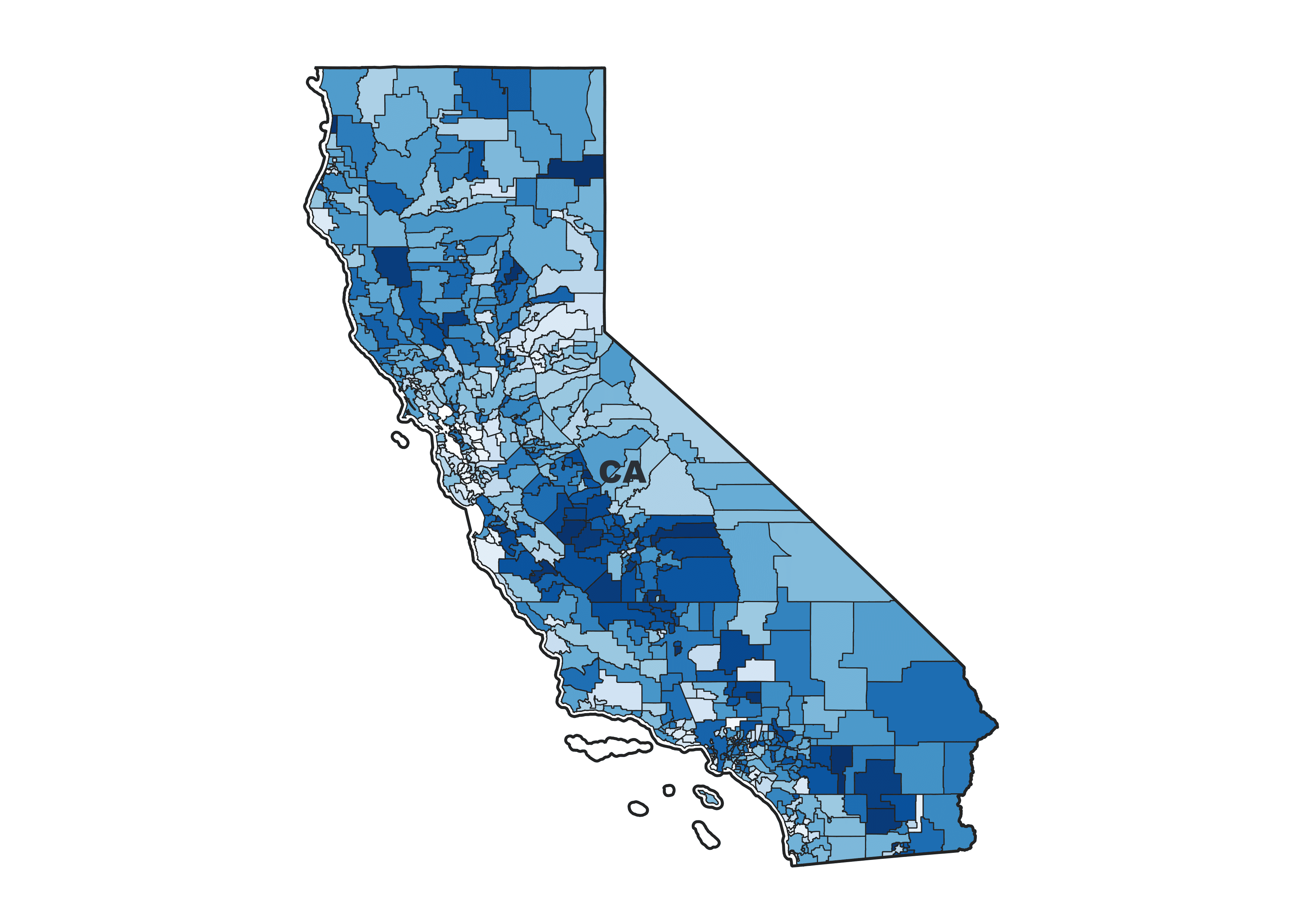
Figure 3b. Students (Economically Disadvantaged)
Figure 3b. Students (Economically Disadvantaged)
Figure 3b. Students (Economically Disadvantaged)
Note: Figures 3a and 3b show the proportions of total number of students (Figure 3a) and the subset of economically disadvantaged students (Figure 3b).
Note: Figures 3a and 3b show the proportions of total number of students (Figure 3a) and the subset of economically disadvantaged students (Figure 3b).
Note: Figures 3a and 3b show the proportions of total number of students (Figure 3a) and the subset of economically disadvantaged students (Figure 3b).
Figures 4a & 4b & 4c & 4d.
Height Extraction
Figures 4a & 4b & 4c & 4d.
Height Extraction
Figures 4a & 4b & 4c & 4d.
Height Extraction

Figure 4a. LiDAR (Los Angeles)
Figure 4a. LiDAR (Los Angeles)
Figure 4a. LiDAR (Los Angeles)

Figure 4b. LiDAR Ground (Los Angeles)
Figure 4b. LiDAR Ground (Los Angeles)
Figure 4b. LiDAR Ground (Los Angeles)

Figure 4c. Height (Los Angeles)
Figure 4c. Height (Los Angeles)
Figure 4c. Height (Los Angeles)

Figure 4d. Height with Building Footprint (Los Angeles)
Figure 4d. Height with Building Footprint (Los Angeles)
Figure 4d. Height with Building Footprint (Los Angeles)
Note: Figures 4a, 4b, 4c, and 4d describe the height extraction process using LiDAR.
Note: Figures 4a, 4b, 4c, and 4d describe the height extraction process using LiDAR.
Note: Figures 4a, 4b, 4c, and 4d describe the height extraction process using LiDAR.
Figures 5a & 5b & 5c.
Residential Density Plots
Figures 5a & 5b & 5c.
Residential Density Plots
Figures 5a & 5b & 5c.
Residential Density Plots

Figure 5a. Residential Density (Los Angeles)
Figure 5a. Residential Density (Los Angeles)
Figure 5a. Residential Density (Los Angeles)

Figure 5b. Building Footprints (Los Angeles)
Figure 5b. Building Footprints (Los Angeles)
Figure 5b. Building Footprints (Los Angeles)

Figure 5c. Building Footprint with Height Information (Los Angeles)
Figure 5c. Building Footprint with Height Information (Los Angeles)
Figure 5c. Building Footprint with Height Information (Los Angeles)
Note: Figures 5a, 5b, and 5c describe the residential density extraction process using spatial information and a machine learning model.
Note: Figures 5a, 5b, and 5c describe the residential density extraction process using spatial information and a machine learning model.
Note: Figures 5a, 5b, and 5c describe the residential density extraction process using spatial information and a machine learning model.
Figures 5a & 5b.
California Land Use Classification and Development
Figures 5a & 5b.
California Land Use Classification and Development
Figures 5a & 5b.
California Land Use Classification and Development
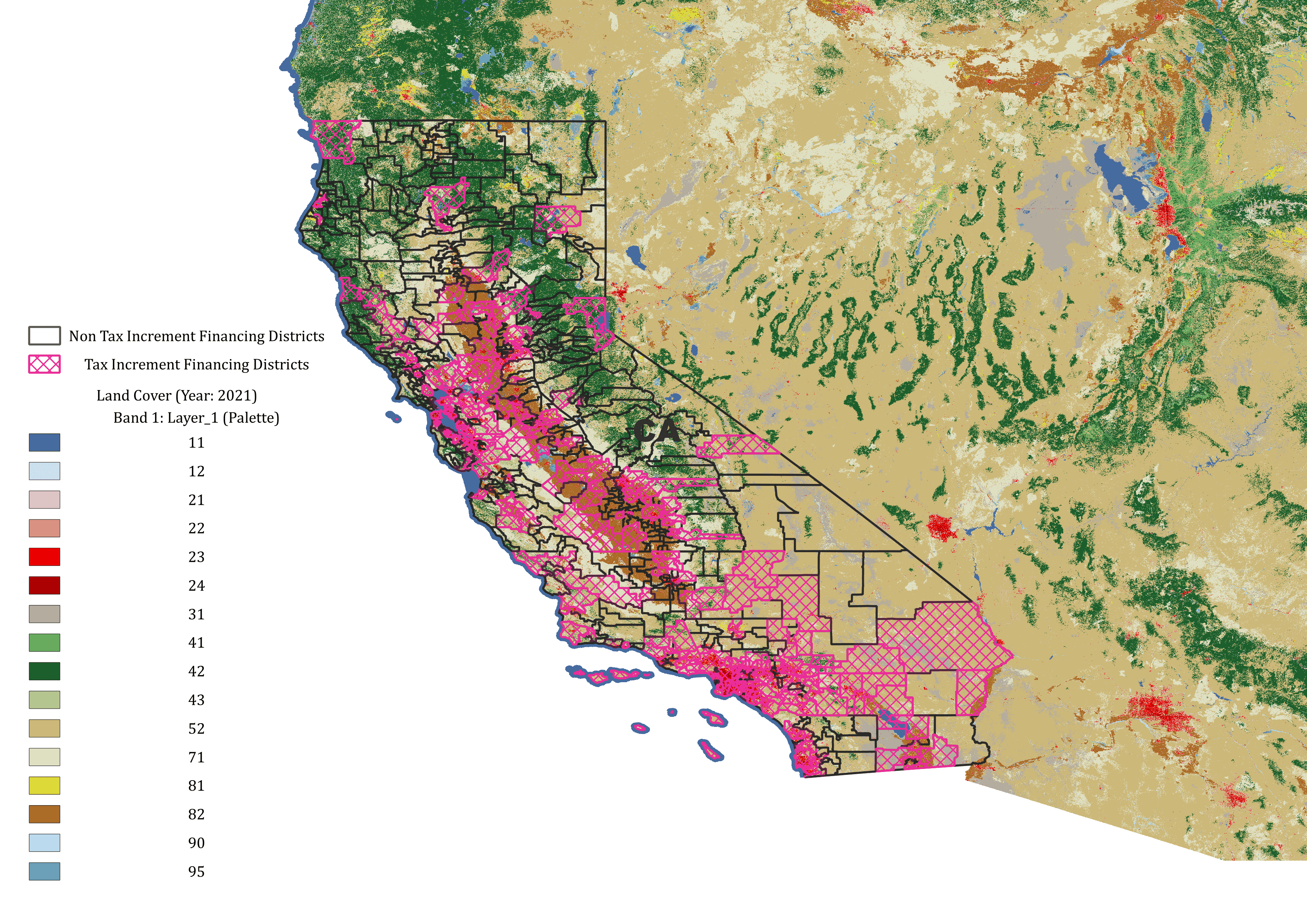
Figure 5a. Land Use
Figure 5a. Land Use
Figure 5a. Land Use
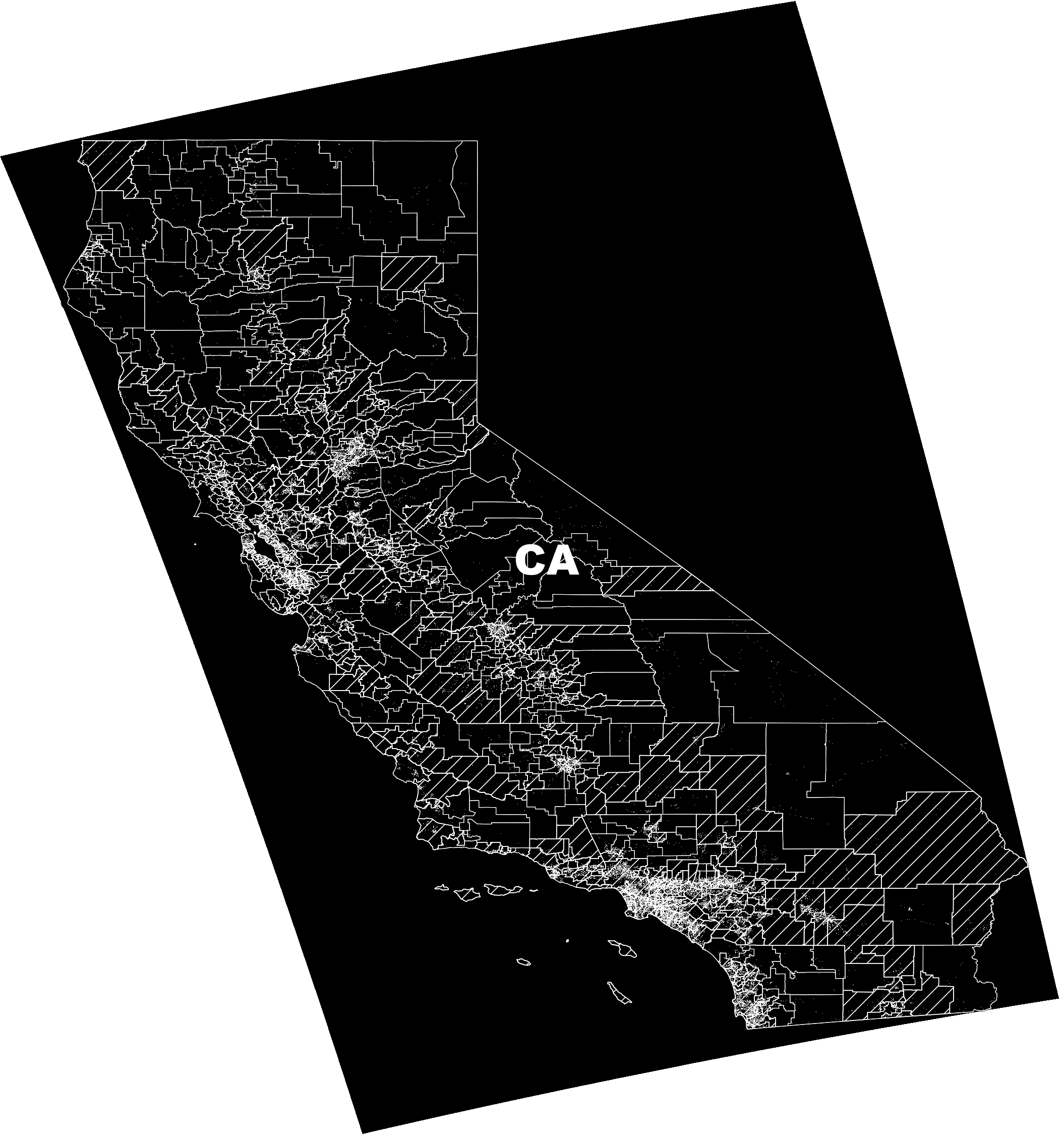
Figure 5b. Modestly Developed Parcels
Figure 5b. Modestly Developed Parcels
Figure 5b. Modestly Developed Parcels
Note: Figures 3a and 3b show the land use classifications (Figure 3a) and the parcels extracted specifically for moderately developed areas (Figure 3b).
Note: Figures 3a and 3b show the land use classifications (Figure 3a) and the parcels extracted specifically for moderately developed areas (Figure 3b).
Note: Figures 3a and 3b show the land use classifications (Figure 3a) and the parcels extracted specifically for moderately developed areas (Figure 3b).
Figures 6a & 6b.
State Education Expenditure by Categories
Figures 6a & 6b.
State Education Expenditure by Categories
Figures 6a & 6b.
State Education Expenditure by Categories
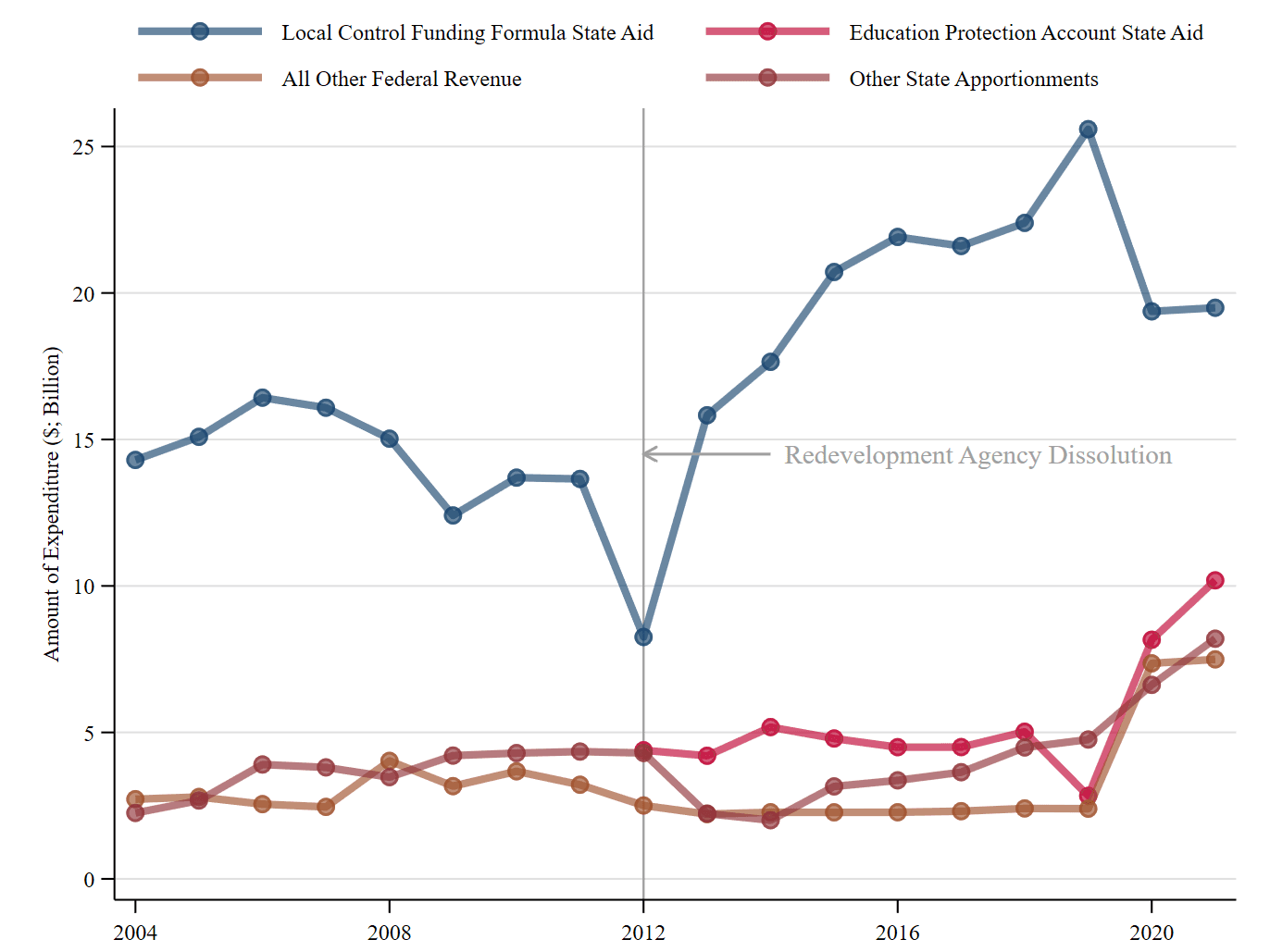
Figure 6a. Tax Increment Financing School Districts
Figure 6a. Tax Increment Financing School Districts
Figure 6a. Tax Increment Financing School Districts
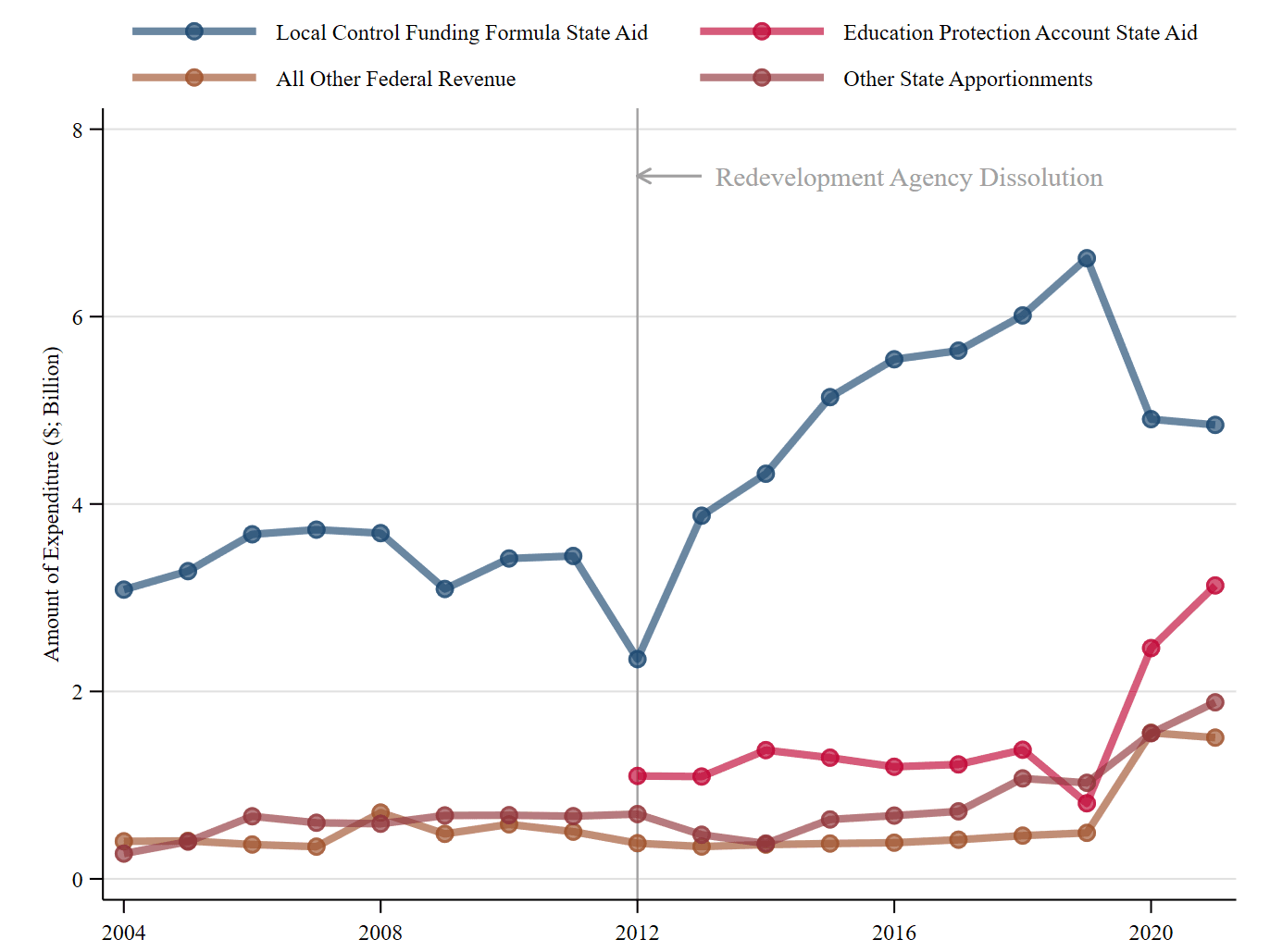
Figure 6b. Non-Tax Increment Financing School Districts
Figure 6b. Non-Tax Increment Financing School Districts
Figure 6b. Non-Tax Increment Financing School Districts
Note: Figures 4a and 4b show state-level education expenditures for school districts that used tax increment financing (Figure 4a) and those that did not (Figure 4b).
Note: Figures 4a and 4b show state-level education expenditures for school districts that used tax increment financing (Figure 4a) and those that did not (Figure 4b).
Note: Figures 4a and 4b show state-level education expenditures for school districts that used tax increment financing (Figure 4a) and those that did not (Figure 4b).
Figures 7a, 7b, & 7c.
Empirical Identification Strategy (Preliminary Results)
Figures 7a, 7b, & 7c.
Empirical Identification Strategy (Preliminary Results)
Figures 7a, 7b, & 7c.
Empirical Identification Strategy (Preliminary Results)
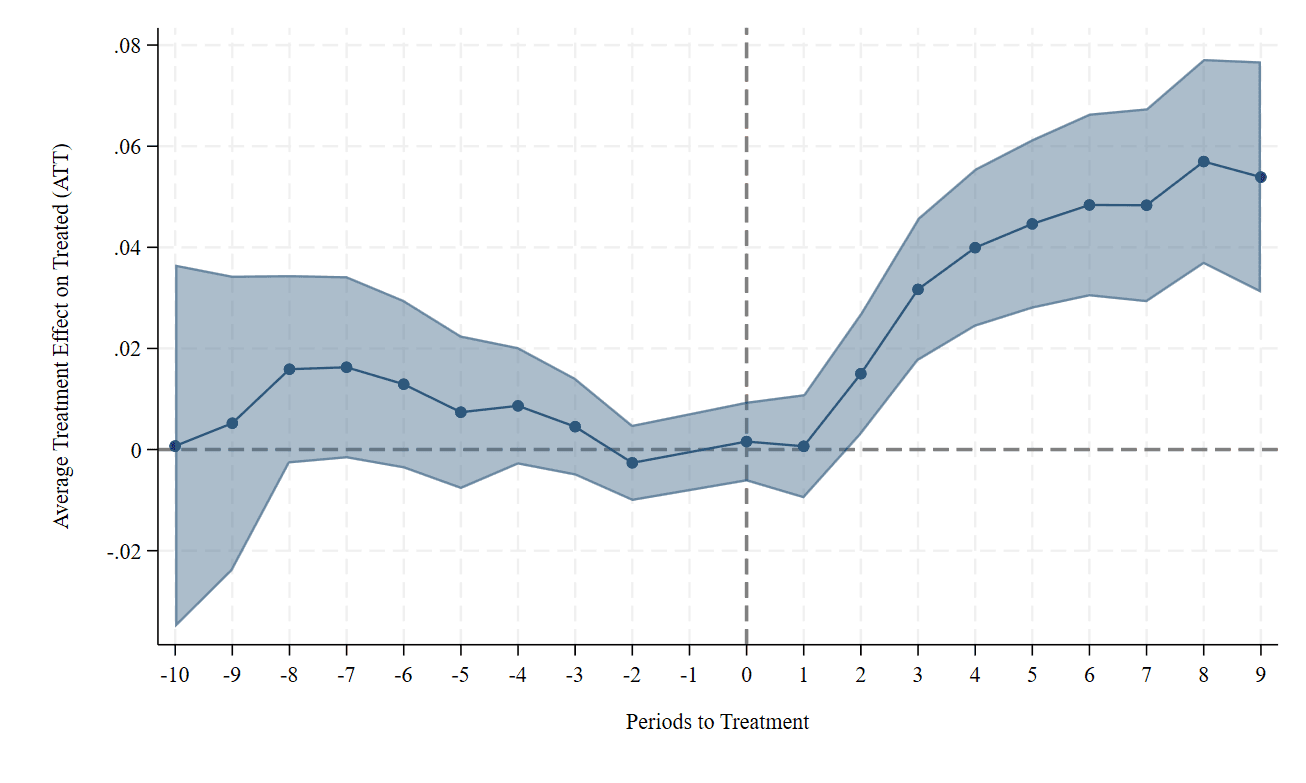
Figure 7a. Two-way Fixed Effect
Figure 7a. Two-way Fixed Effect
Figure 7a. Two-way Fixed Effect
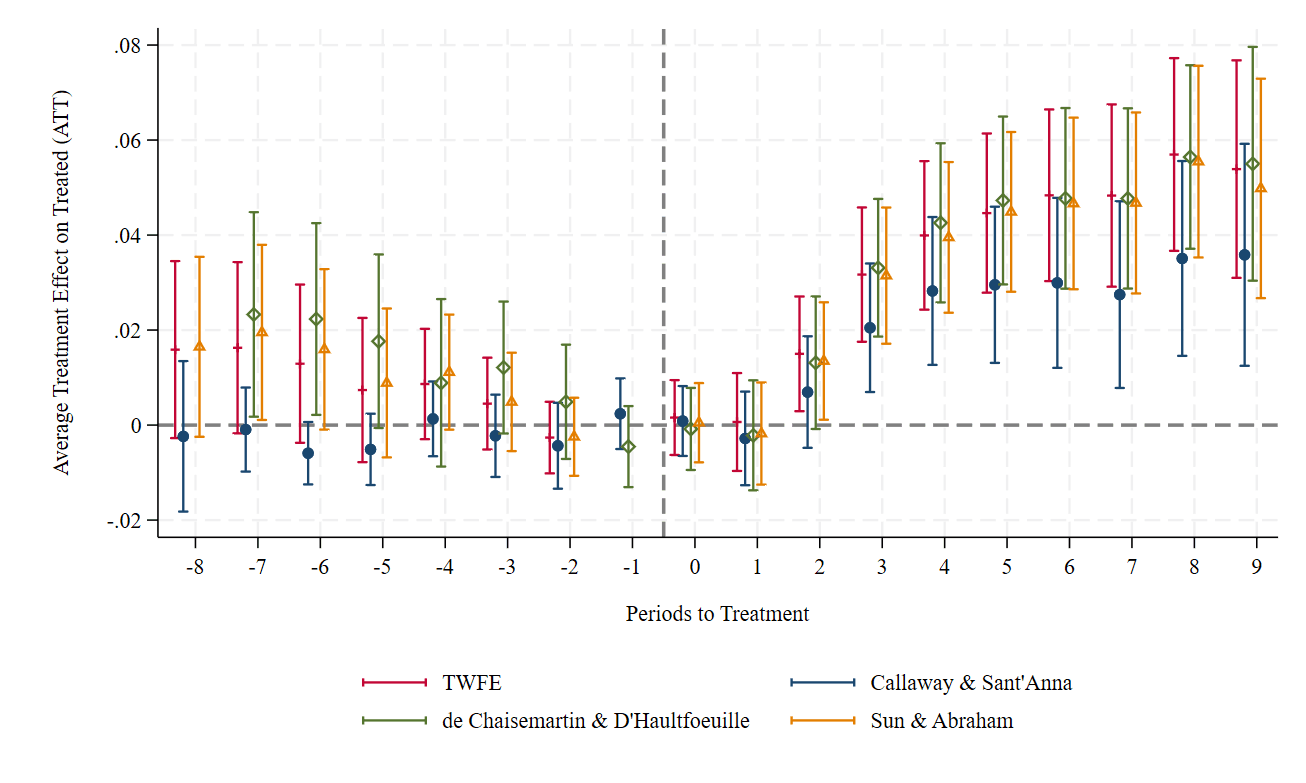
Figure 7b. Sensitivity Analysis
Figure 7b. Sensitivity Analysis
Figure 7b. Sensitivity Analysis
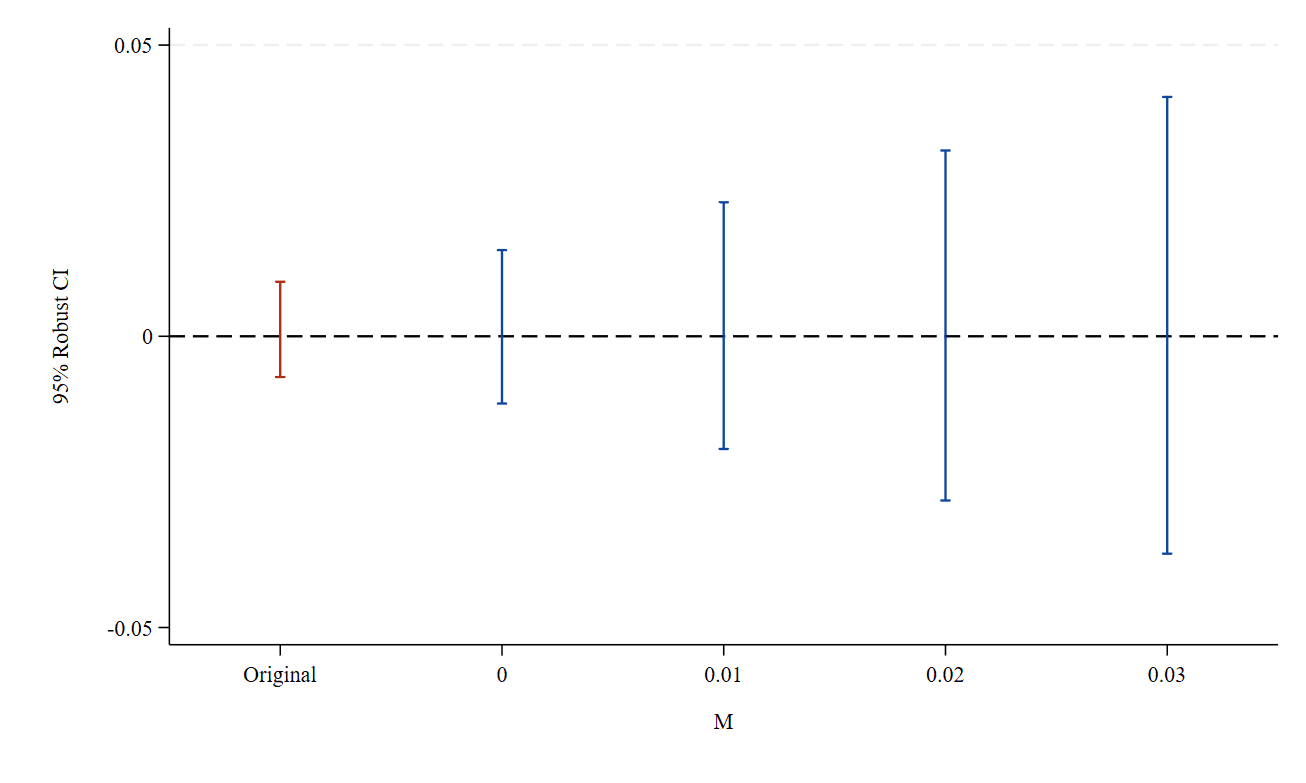
Figure 8c. Bound Test (Rambachan & Roth, 2022)
Figure 8c. Bound Test (Rambachan & Roth, 2022)
Figure 8c. Bound Test (Rambachan & Roth, 2022)
Note: Figure 5a evaluates the impact of the dissolution of California's redevelopment agencies using a two-way fixed effects model. Figure 5b conducts a sensitivity analysis with various identification strategies for difference-in-differences (DiD) analysis. This part includes the approach of Callaway and Sant'Anna (2021), which uses propensity score-weighted DiD to match treatment and control groups with similar characteristics. Note that this analysis uses a code developed by Borusyak (2023). Figure 5c examines the violation of the pre-trend using the methodology of Rambachan and Roth (2022).
Note: Figure 5a evaluates the impact of the dissolution of California's redevelopment agencies using a two-way fixed effects model. Figure 5b conducts a sensitivity analysis with various identification strategies for difference-in-differences (DiD) analysis. This part includes the approach of Callaway and Sant'Anna (2021), which uses propensity score-weighted DiD to match treatment and control groups with similar characteristics. Note that this analysis uses a code developed by Borusyak (2023). Figure 5c examines the violation of the pre-trend using the methodology of Rambachan and Roth (2022).
Note: Figure 5a evaluates the impact of the dissolution of California's redevelopment agencies using a two-way fixed effects model. Figure 5b conducts a sensitivity analysis with various identification strategies for difference-in-differences (DiD) analysis. This part includes the approach of Callaway and Sant'Anna (2021), which uses propensity score-weighted DiD to match treatment and control groups with similar characteristics. Note that this analysis uses a code developed by Borusyak (2023). Figure 5c examines the violation of the pre-trend using the methodology of Rambachan and Roth (2022).
Table 1.
Summary Statistics
Table 1.
Summary Statistics
Table 1.
Summary Statistics
Note: This table present the summary table of selected variables at the school district level for the education expenditure per pupil. "Count," "Mean," "SD," "Min," and "Max" are the number of observations, the sample mean, the standard deviation, and the minimum and maximum values, respectively.
Note: This table present the summary table of selected variables at the school district level for the education expenditure per pupil. "Count," "Mean," "SD," "Min," and "Max" are the number of observations, the sample mean, the standard deviation, and the minimum and maximum values, respectively.
Note: This table present the summary table of selected variables at the school district level for the education expenditure per pupil. "Count," "Mean," "SD," "Min," and "Max" are the number of observations, the sample mean, the standard deviation, and the minimum and maximum values, respectively.
Conclusion
Conclusion
Conclusion
Work-in-progress.
Work-in-progress.
Work-in-progress.
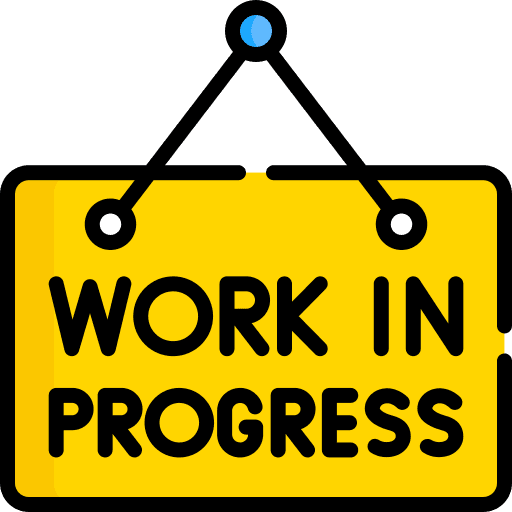
© 2025 WOOSERKPARK.COM. All rights reserved
© 2025 WOOSERKPARK.COM. All rights reserved